Thinking slow, fast, exponential: is AGI really here?
- Massimiliano Turazzini
- Feb 9
- 8 min read
Last week, Alberto Romero wrote a thought-provoking post titled "AGI Is Already Here—It’s Just Not Evenly Distributed". With great simplicity, he summarized the opportunities AI offers 'at different levels of use.' After reading my post, I invite you to spare 10 minutes for his insights.
In essence, he says that the mere growth of AI is an ample advantage to enhance our 'performance' with the new models, even if we don't know how to write prompts. However, we can further elevate our capabilities with the same technology when we know how to use them — to craft prompts effectively and interact proficiently with THAT model.
That article got me thinking a lot, so I tried to reinterpret it my way, sharing the bulbs that lit up for me because we are, as always, facing something very, very impactful.
Spoiler for both: We are not yet talking about Artificial General Intelligence, but after reading this post, you'll understand why it is being mentioned more and more often.
My Recent Thoughts
I have been sharing vast amounts of information and receiving even more. There is no respite in the evolution of AI and no time to keep up with everything. As I often say, it's not a technology problem (which is becoming more complex and harder to understand) but an issue of the impact on everything we once considered expected.
Fundamentally, it used to be expected for a person to gain education in their early years and continue learning thereafter at more or less regular paces, with some accelerations here and there provided by school, being in the right environments, having the right attitude, and the necessary insights to improve their 'performance.' (Permit me to use 'performance' in this post as a reductive term to avoid speaking of intelligence, to include concepts of efficiency, satisfaction in execution, related emotions, success, etc.)
Then, around 2017, I started encountering the first Language Models and realized that the concept of normal would change quickly. I wrote a novel that took me over a year of work: "Glimpse," to take a look at what could happen with AI systems capable of understanding, comprehending, and consequently responding to us humans, regardless of the technology itself. I won't bore you with my recent history, but in this journey with AI I am discovering more things than I will ever be able to dig into.
For example:
The mistake of delegating to AI tasks that could prove harmful for us.
We should always improve after each interaction with an AI, either directly (new knowledge) or indirectly (saving time on mundane tasks to do something more valuable).
The acceleration is continuous, with strong jolts, making us quickly leave the path we were on to get on an even steeper one
AI is fast, but we need time to assimilate it.
We will never learn to do everything well.
Every three years, I probably had to unlearn things, now maybe every three months (For instance, before this post, it seemed sensible to unlearn how to craft a good prompt, but as you will understand, this is not the case)
I used to measure time in terms of the number of times technology amazed me in a year. Now "Aha moments" are trending, and I used to call them 'eureka moments,' but you get the idea. There were periods with zero such moments per year. Recently, I've reached even three moments of astonishment a week.
Some "Aha Moments"
There are times when our awareness increases and our growth follows suit, moments when we become aware of an impending or already occurred change and realize that we will have to deal with it.
One such moment happened while reading Alberto Romero's post, which gathered a series of other "Aha Moments" I’ve had over the years and wonderfully aligned them in my head, which is now about to explode.
In no particular order:
The book "Exponential Organizations" by Salim Ismail
The first LLMs that started to give sensible responses (GPT 3.0)
The first LLMs that began to understand structured and very complex requests (GPT 4.0)
The first multimodal models and all the series of evolutions in recent years in models from different producers
The first LLMs that started to 'act' with other software (GPT4 with code interpreter)
The distilled SLMs (if you don’t know what they are... here in Italian and here in English ChatGPT explains it quickly)
The scaling laws of AI, training, inference, reasoning (I will have to write something simple about this, I won’t delegate a rushed response to ChatGPT)
Understanding our role when interacting with an AI (here’s Ethan Mollick’s initial vision)
The concept of instrumental delegation and cognitive delegation, which I discuss here (here in English)
The concept of Jolting Technology https://www.linkedin.com/pulse/jolting-technologies-david-orban/
Reasoning Models (the O1, O3 series, Deepseek, Gemini Flash Thinking), models that think before answering.
AI agents like Cline and Lovable that develop an entire software from an analysis and a few prompts for me
The Reasoning Agents: models that not only think but also have tools at their disposal, as I explain here and here in English
Seeing various AI agents interact with each other in agent-specific systems
The 'nested boxes' game of exponential growth is wonderfully explained by Peter Gostev here.
And a bunch of other interesting information I studied over the years.
I know, there are quite a few points, but the theme here is the complexity combined with a flurry of rapid evolutions that make finding precise figures by connecting the dots challenging.
And I confess that in my current journey with AI, I often have a lot of doubts and perplexities (I don’t want to call them fears) about what will impact, how it will affect, how we will adjust to this rapid pace of evolution, what will happen when we make the presence of AI normal, which will continue to grow, in our lives.
Will normalizing coexistence with AI mean entering into a symbiosis? And what will happen if there are many shocks, many points of discontinuity, if, in the course of this symbiosis, one of the two partners runs and evolves too fast? But I digress, let's get back to today.
Augmented Intelligence
Apologies as always to all the graphic designers, but this is my revised and generalized representation of Alberto Romero’s chart.
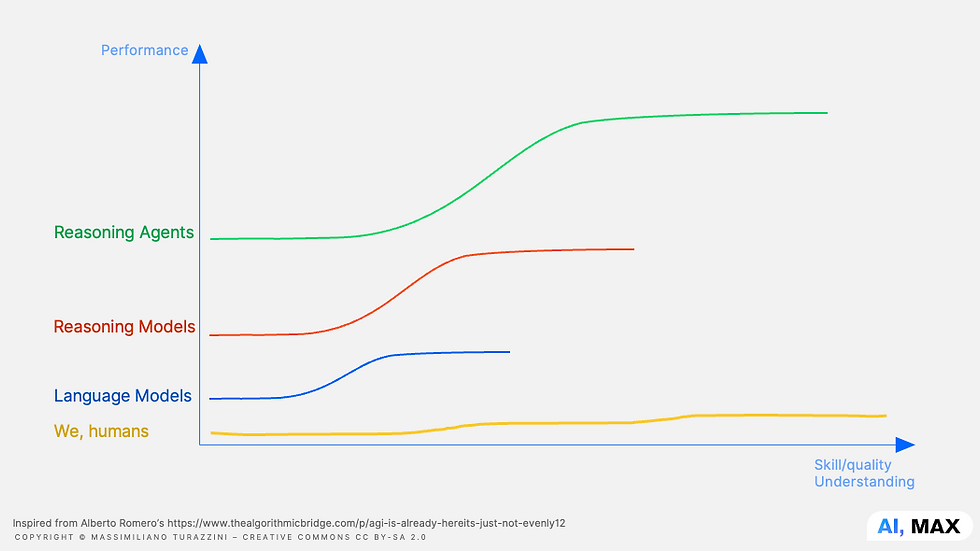
In his case, he talks explicitly about prompting skills and some models from OpenAI. I'm attempting to generalize it.
So on the X-axis, you find our skills as humans, the quality with which we express ourselves and use a tool with which we understand.
On the Y-axis, 'performance' as I described at the beginning of this post. Performance can now be measured by having a sort of Amplified Intelligence (which I like much more than Artificial Intelligence) at our disposal. AI as an amplifier of our abilities.
Here, you won’t find units of measurement, and the scales and distances are probably different. Still, we are interested in the distance between the different curves (I always get a shiver when it comes to numerically measuring a mental performance).
I have also added the series "we humans," which constantly and inexorably improve over time, both as individuals and as a species, albeit at an evolutionary, indeed not exponential, pace.
In Practice
But what happens when we start using Language Models? Not knowing how to use it (writing bad or random prompts) allows us to make a leap forward in our performance because the model itself increases our chances of better performance.
If we then begin to understand it and know how to use it (by writing better prompts), then the qualitative leap, model being equal, increases a lot.
Reasoning models, in themselves, offer a greater quality leap than someone who has perfect mastery of a basic model would achieve. In other words, even if you are an expert at prompting with GPT-4o, the inherent capabilities of a reasoning model (such as O1) surpass those of a traditional model, even with a simple prompt.
The third stage occurs with Reasoning Agents, i.e., when we give Reasoning Models tools (e.g., Web search, knowledge base, private data) and let them explore the information themselves. Here, the prompts become much more complex to maintain control, but if you want to let them (the eye) do it, just talk to them like an apprentice.
For example, the first time I tried out O1-Pro with DeepSearch, I had one of the biggest "aha" moments of my life....
Why? Read the next paragraph and take a deep look at the picture.
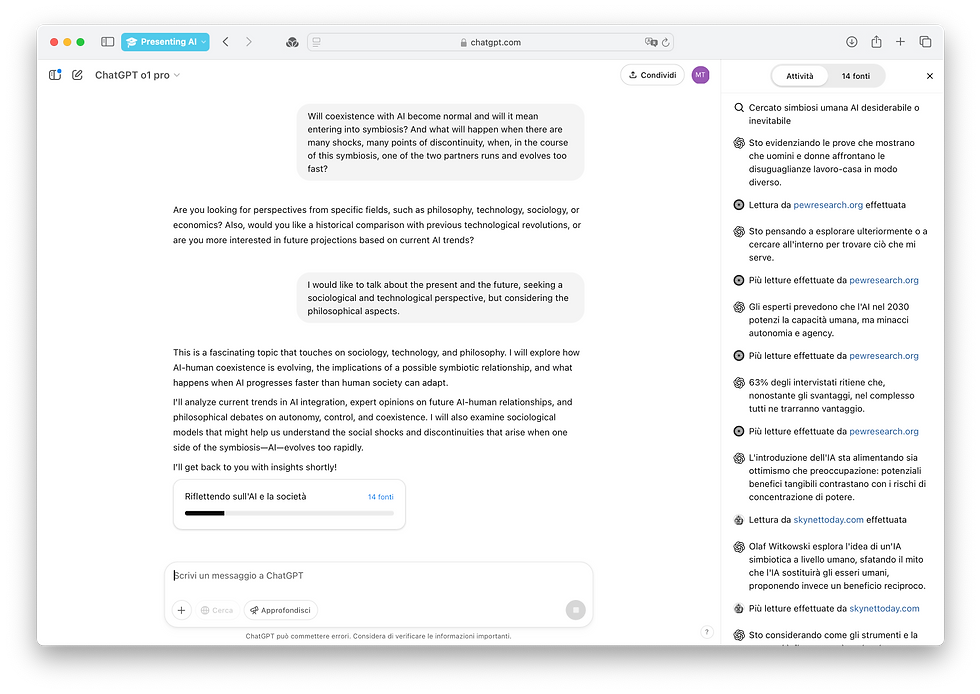
I simply reported the paragraph above about symbiosis, leaving 'him' all the subsequent research. Seeing an AI agent go deep into concepts and take care about finding information, reading them one by one, adapting its following strategies based on what it has previously learned, re-reading and autonomously reassessing... well, it’s quite an effect. Here you find the conversation in Italian and here in English.
If You Listening to Everyone...
If we then put together all the concepts, all the capabilities of the various models, the growth of computational power incessantly recounted by Jensen Huang, CEO of NVidia, Kurzweil’s prophecies from Singularity University, and David Orban’s Jolting Moments... we obtain something like this.

Those who know how to exploit these tools may evolve at speeds that once required millions of years.
Leaving those who lag behind to wonder where everyone has gone.
I don't want to create further FOMO (Fear Of Missing Out, the fear of being left out) with this exponential growth, but simply recount that this is the direction humanity is taking, whether we like it or not. As recounted in the post by Alberto Romero, there is a problem of fair distribution that we already know and will further increase.
So what...
The rule of "Knowing how to ask questions," to simplify the concept of prompting, continues to hold. Be careful; this doesn’t mean that a prompt done well in 4o works equally well in O1 with Deep Research, actually, quite the opposite. Each model has capabilities, limits, and quirks and must be explored. Each model requires constant time and testing to verify its effectiveness.
The exact mechanism will apply in many other fields. Only if we do it as has happened over the last ten thousand years, the growing times will be very extended.
If we are constantly stimulated and challenged by more potent and faster AI models, we have the opportunity to greatly and quickly amplify our capabilities, often going beyond our understanding.
If we lean on the minimum capabilities of AI models, we will still have the chance to improve our performance very quickly. Knowing how to use them well, what the future holds for us, we can also amplify ourselves and perhaps follow this increasingly rapid acceleration.
Perhaps it is now time for Daniel Kahneman to make a new edition of his book, 'Thinking, Fast and Slow.' The new title could be: 'Thinking, Fast, Slow and Exponential,' and it might help us understand this evolution in which we are already immersed.
Moreover, in the meantime, we should understand how to remain human. How do we preserve our critical thinking, stay empathetic, and make decisions based on our values, knowledge, and instincts rather than relying on these often inhuman growth curves? How can we give these tools the right level of agency while remaining creative and in control? How do we distribute this Augmented Intelligence fairly?
Enjoy AI responsibly!
Massimiliano
If you liked this article, share it, you will help me write more 😀
Learn more on Linkedin or on https://maxturazzini.com.
Yorumlar